Bayesian Inference in Dynamic Econometric Models: A Comprehensive Guide

Bayesian inference has emerged as a powerful approach for analyzing dynamic econometric models due to its ability to incorporate prior information and account for uncertainty in model parameters. This article provides a comprehensive overview of Bayesian inference in dynamic econometric models, covering the theoretical foundations, practical implementation, and applications of Bayesian methods in this field.
4.7 out of 5
Language | : | English |
File size | : | 4527 KB |
Text-to-Speech | : | Enabled |
Print length | : | 376 pages |
Lending | : | Enabled |
Bayesian Inference: Fundamentals
Bayesian inference is a statistical approach that utilizes Bayes' theorem to update beliefs about unknown parameters based on observed data. It begins with a prior distribution, which represents the initial beliefs about the parameters, and a likelihood function, which describes the probability of the observed data given the parameters. Bayes' theorem then combines these to compute the posterior distribution, which represents the updated beliefs after considering the data.
In dynamic econometric models, the parameters often evolve over time. Bayesian inference allows for the estimation of these time-varying parameters, providing insights into the underlying dynamics of the system being studied.
Markov Chain Monte Carlo (MCMC) Methods
Markov chain Monte Carlo (MCMC) methods are a family of computational techniques used to approximate the posterior distribution in Bayesian inference. These methods involve simulating a Markov chain that converges to the target distribution, allowing for the generation of samples from the posterior.
Popular MCMC algorithms include the Metropolis-Hastings algorithm, the Gibbs sampler, and the Hamiltonian Monte Carlo (HMC) algorithm. The choice of MCMC algorithm depends on the complexity of the model and the desired computational efficiency.
Applications of Bayesian Inference in Dynamic Econometrics
Bayesian inference has found广泛的应用in dynamic econometrics, including:
- Time Series Analysis: Estimating and forecasting time-varying parameters in time series models, capturing trends, seasonality, and volatility.
- Panel Data Analysis: Analyzing dynamic relationships between cross-sectional units over time, accounting for unobserved heterogeneity and time dependence.
- State Space Models: Estimating hidden states and parameters in models where only indirect observations are available, such as in Kalman filtering and smoothing applications.
- Non-Gaussian Models: Handling non-Gaussian error distributions, allowing for more flexible and realistic modeling of economic phenomena.
- Model Selection and Hypothesis Testing: Using Bayesian methods to compare alternative models and test hypotheses, providing robust and informative results.
Challenges and Best Practices
While Bayesian inference offers powerful advantages, it also presents some challenges:
- Computational Complexity: MCMC simulations can be computationally intensive, especially for complex models.
- Model Sensitivity: Bayesian results can be sensitive to the choice of prior distributions and MCMC algorithms.
- Interpretability: Communicating Bayesian results can be challenging due to the inherent uncertainty and probabilistic nature of the inferences.
Best practices for mitigating these challenges include:
- Efficient MCMC Algorithms: Selecting and tuning MCMC algorithms to optimize computational efficiency.
- Robust Sensitivity Analysis: Checking the stability of results under different prior distributions and MCMC settings.
- Clear Communication: Providing clear explanations of Bayesian concepts and results, emphasizing the probabilistic nature of the inferences.
Bayesian inference has revolutionized dynamic econometric modeling by enabling the incorporation of prior information, estimation of time-varying parameters, and handling of complex error distributions. With the advent of powerful computational tools and best practices, Bayesian methods have become increasingly accessible and applicable in a wide range of economic research areas.
By understanding the theoretical foundations, practical implementation, and challenges associated with Bayesian inference in dynamic econometric models, researchers can leverage this powerful approach to gain deeper insights into economic phenomena and make informed decisions based on reliable statistical analyses.
References
- Gamerman, D., & Lopes, H. F. (2006). Markov chain Monte Carlo: Stochastic simulation for Bayesian inference. Chapman and Hall/CRC.
- Gelman, A., Carlin, J. B., Stern, H. S., Dunson, D. B., Vehtari, A., & Rubin, D. B. (2014). Bayesian data analysis. Chapman and Hall/CRC.
- Kim, C.-J., & Nelson, C. R. (1999). State-space models with regime switching: Classical and Bayesian methods and applications. MIT press.
4.7 out of 5
Language | : | English |
File size | : | 4527 KB |
Text-to-Speech | : | Enabled |
Print length | : | 376 pages |
Lending | : | Enabled |
Do you want to contribute by writing guest posts on this blog?
Please contact us and send us a resume of previous articles that you have written.
Best Book Source
Ebook Universe
Read Ebook Now
Digital Book Hub
Ebooks Online Stores
Fiction
Non Fiction
Romance
Mystery
Thriller
SciFi
Fantasy
Horror
Biography
Selfhelp
Business
History
Classics
Poetry
Childrens
Young Adult
Educational
Cooking
Travel
Lifestyle
Spirituality
Health
Fitness
Technology
Science
Arts
Crafts
DIY
Gardening
Petcare
Robert Beardsley
Samir Chopra
Marly Cornell
Dave Batista
John Conroy
Philip Bowring
Sidney Dekker
Jane Goodall
Margarita Nelipa
Flora Gregg Iliff
Irenosen Okojie
David Trout Pomeroy
Rob Beckett
Karen Woods Weierman
Susan F Hirsch
Harvey Fierstein
Ramon Ray
John James Santangelo Phd
Elle Andra Warner
Neil Malhotra
Light bulbAdvertise smarter! Our strategic ad space ensures maximum exposure. Reserve your spot today!
- Doug PriceFollow ·3.3k
- Adrien BlairFollow ·17k
- Paulo CoelhoFollow ·6.6k
- Brian BellFollow ·7.5k
- Benjamin StoneFollow ·2.9k
- Isaac MitchellFollow ·5.2k
- Gerald BellFollow ·8.8k
- Stanley BellFollow ·6.8k
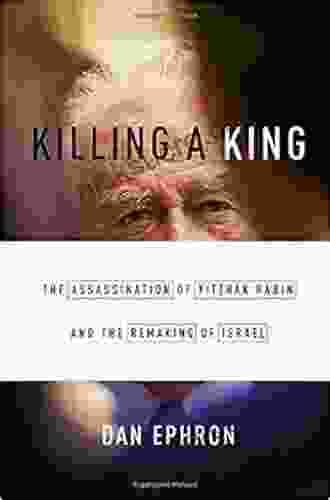

Killing A King: The Assassination Of Yitzhak Rabin And...
## The Assassination Of Yitzhak Rabin And The...
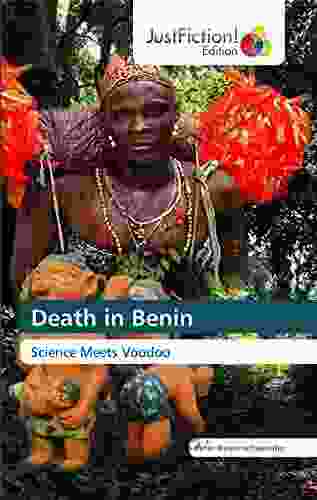

Death in Benin: Where Science Meets Voodoo
In the West African nation of Benin, death...
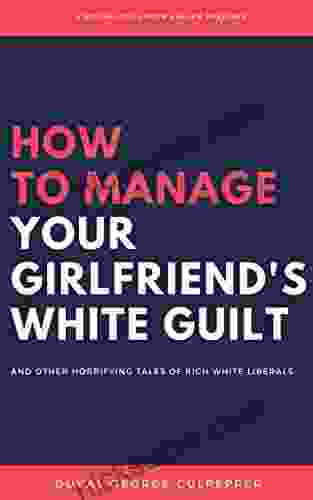

A Comprehensive Guide to Managing Your Girlfriend's White...
White guilt, a complex and...
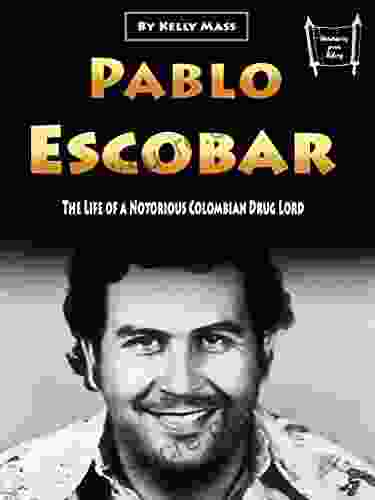

The Notorious Life and Times of Pablo Escobar, the...
Pablo Escobar, the...
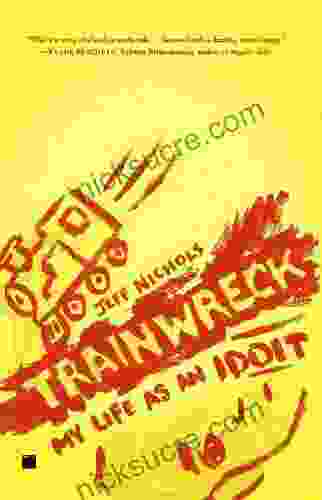

Trainwreck: My Life As An Idiot
My life has been a trainwreck. I've made...
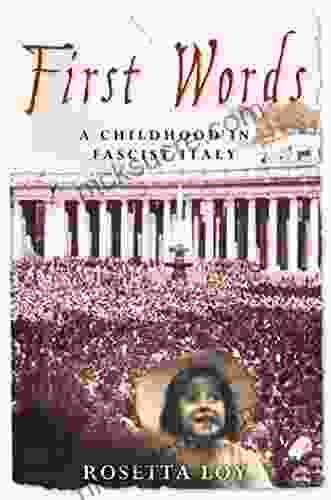

First Words Childhood In Fascist Italy: A Haunting Memoir...
First Words Childhood In...
4.7 out of 5
Language | : | English |
File size | : | 4527 KB |
Text-to-Speech | : | Enabled |
Print length | : | 376 pages |
Lending | : | Enabled |